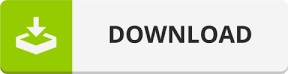
Despite their name these vectors can be accessed and mutated from the host as well as the device. The data is stored in device_vectors which are initialized, and can be interacted with much like std::vector objects. Executions of the returned function will return a random number sample from a uniform distribution. The first call sets the bounds for the random floats. In a JavaScript context I would think of this as a second order function. The custom kernel operation initRandomPrg is organized as a struct that implements the () operator, meaning it can be executed after initialization. This allowed my final Makefile to be fairly simple. However, because Thrust is included as part of the Cuda toolkit, I don’t need to link any external libraries. There were a couple of gotchas I ran into. I’m running Ubuntu 18.04, using the nvidia-driver-390 metapackage. My computer has a Nvidia GTX 1060 (6GB) graphics card. In this post I take a look at creating a simple program using Cuda’s core library, Thrust, a serialized version. As we’ll see this makes it a little bit easier to work with.
#Cuda very expensive cudalaunch calls drivers#
The Cuda drivers and libraries can be installed by apt-get, I didn’t have to build a kernel image, and the Thrust library is now included with the Cuda toolkit. While things aren’t perfect - the driver packages still don’t play very nicely with each other - there has been a marked improvement. While I’ve been intrigued, for a while the hardware was difficult to get access to or the libraries were difficult to use.
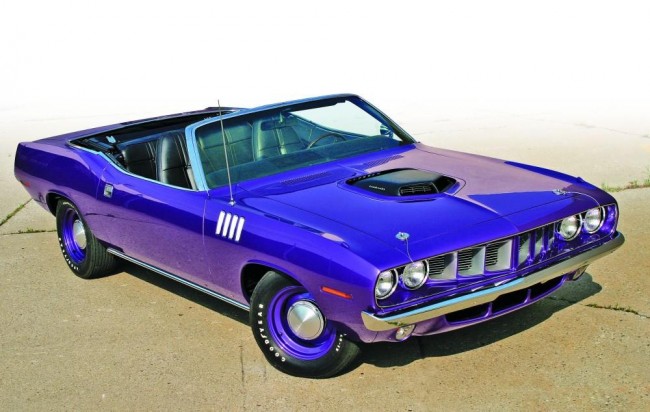
I took a course during my undergrad as well as the Udacity offering a few years ago. I have a limited background in Parallel programming.
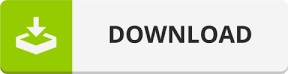